Minimum quantity (MQ) is popular among traders as a tool for improving execution quality. As an exchange, we have a unique perspective on the effectiveness of MQ use. In this three-part series, we attempt to quantify how effective MQ use is in optimizing execution.
There are many factors that impact execution quality — a few of them are adverse selection, information leakage, and ability to source liquidity. The weight that is applied to each of these changes depending on the goals of the trader and the strategy.
In Part I, our analysis on IEX found that MQ may not be very effective for mitigating adverse selection. In Part II, we dive into MQ’s ability to minimize information leakage.
First, we look at whether fewer trades over the life of an order correlates with improved performance, consistent with conventional wisdom. Second, we try to determine if larger MQs are likely to be an effective tool for reducing the number of trades needed to fill an order.[1]
In short, Part II utilizes data from shares traded on IEX since the beginning of 2020 to attempt to answer the question: Can using MQ to trade a large order in fewer trades improve execution quality?
Information Leakage Today
Every trade produces a footprint of information that is visible to the market, so filling an order in fewer trades should “leak” less information. This information is generated because all trades are reported to the tape and disseminated via market data feeds. This mechanism, though important for transparency and an efficient market, allows participants to glean information about orders and attempt to profile market participants. The value of this information varies by strategy, but we will provide two examples of how giving information up to the market can negatively impact your performance.
In these examples, you are a buyer of 1,000 shares of XYZ stock. You trade 300 shares at $10.01. This trade of 300 shares at $10.01 is printed to the tape and disseminated via market data feeds.
Ex 1. There is another buyer of 200 shares of XYZ who sees your 300-share trade print. The other buyer now knows there is competition in the market and gets more aggressive by bidding at a higher price, hoping they get the fill before you do. Their aggressive behavior drives the stock price up and you now buy your remaining XYZ for more than $10.01.
Ex 2. The counterparty that sold you 300 shares of XYZ only has 100 shares left to sell of their order. After trading 300 shares with you, they know that there was a buyer of at least 300 shares at $10.01. The seller assumes that you have more to buy and waits for you to push the stock up to a price more favorable for them (the seller). The retreat of the seller from $10.01 means the price of XYZ stock rises and you now must pay more than $10.01.
One way to reduce the number of trades throughout an order is to use a MQ, which increases the size of your trades to a minimum share threshold, thus lowering the number of trades needed to complete your order.
High MQs may help limit information leakage because they ensure that each trade in the order will fill a specified number of shares. However, traders must weigh the trade-offs between the risk associated with waiting around for big counterparties, which may potentially never come (we will explore liquidity risk in Part III of this series), and the information leakage associated with participating in a larger number of trades.
Optimizing Number of Trades Throughout an Order
First, we must provide data around the examples from the previous section to demonstrate that more trades lead to more information leakage. To do this, we look at slippage vs. arrival price of large resting orders [2] as a percent of the stock’s spreadbased on how many trades it took to fully fill an order.
We use slippage vs. arrival as proxy for the impact caused by “leaking” information to the market because it measures the change in a stock’s midpoint price from when the order is entered until the last trade occurs and the order leaves the market. As the market becomes “aware” of a large buyer, it is likely that other buyers will feel the competition and get more aggressive, driving the stock price up, and, on the flip side, sellers may get greedy and wait until the stock price increases before they sell.
As expected, our data suggests slippage is better (slippage closer to 0% or negative) when fewer trades are used to fill an order.
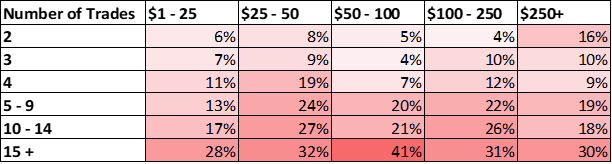
We must also account for order size, as larger parent orders are harder to trade in fewer trades, and thus naturally leak more information. In other words, it is easier to find counterparties large enough to trade a 500-share order in two 250-share trades than it is to find counterparties to trade a 5,000-share order in two 2,500-share trades. Below, we look at slippage vs. arrival bucketed by the order’s median trade size relative to the parent order.
Consistent with our finding that performance improves with fewer trades per order, we find that slippage improves as trade size relative to order size increases. Notably, there is a material difference in slippage when the median trade size is less than 5% of the order size as compared to median trade sizes of more than 5%.

Our evidence shows that on IEX, fewer and larger trades over the life of an order correlates with decreased information leakage and improved performance.
Is a Higher Minimum Quantity the Best Way to Limit Information Leakage?
The optimal way to limit information leakage through fewer trades is to find a large, natural counterparty at a mutual price. However, being in the right place at the right time is notoriously difficult. Given that no one can guarantee perfect timing, traders use tools, like MQs, to try to trade fewer times throughout an order by restricting themselves to a minimum trade size.
If limiting trade size alone helped to improve order performance, we would expect orders with larger minimum trade sizes to outperform orders with smaller minimum trade sizes. Said simply, the larger the minimum trade size, the better the performance should be.
In the prior section, we expressed trade size as a function of parent order size, which is seldom the way it’s viewed by traders, so to help with real-world applicability we analyzed the minimum trade size throughout the life of an order as a proxy for the MQ values traders could have used for these orders. Looking at slippage vs. arrival data for large orders (1,000–10,000 shares) based on the minimum trade size of the order, we can determine if larger minimum trade sizes perform better than smaller.
We find significant performance gains when the smallest trade size was at least 100 shares in stocks priced over $50, and at least 200 shares for stocks priced under $50. This makes sense as setting a 100 MQ on a stock priced $20 represents a much different notional barrier than a 100 MQ on a stock priced $200. The liquidity dynamics of stocks are partially a function of security price, so minimum trade size should, to some extent, reflect the notional value of the trade vs. just the number of shares.
However, in all price bins we see little performance benefit for minimum trade sizes larger than the 200–299 share bucket. This is significant because if high MQs were an effective tool to limit information leakage, we should see continued improvement as minimum trade size increases.

Larger Minimum Trade Size Does Not Improve Performance
As noted above, our analysis suggests that artificially manufacturing fewer trades on your order by setting high MQs over 100 shares (or 200 shares for stocks <$50) may not improve performance on IEX. Though at the exchange level we do not have perfect information on parent orders, we hope that these findings are useful when considering MQ use to reduce information leakage.
Furthermore, as we found in Part I, using high MQs (1,000+ shares) can increase the likelihood of adverse selection. Though MQ seems to be useful when preventing information leakage by filtering out very small trades, setting high MQs may increase adverse selection without decreasing information leakage.
Additionally, if the largest minimum trades sizes do not show material performance improvement, there does not seem to be a compelling reward to compensate for the risk of limiting the amount liquidity you can interact with by setting high MQs. In Part III, we will analyze the potential risks of missing liquidity when using MQs.
[1] An important methodology note: As an exchange, we don’t have access to parent order data from the broker-dealer’s perspective. For this analysis, we isolated orders between 1,000 and 10,000 shares sent to IEX by Agency and Full Service Broker-Dealer Member firms (IEX classifications are on a best efforts basis by Member firms’ trading sessions) that were fully filled and had no MQ. Of course, this is an imperfect proxy. We welcome conversations with our Member partners if they would like to collaborate on more extensive analysis of this topic.
[2] We looked at fully filled orders between 1,000 and 10,000 shares from the liquidity adder’s perspective.